Master Unsupervised Learning: Future of MI
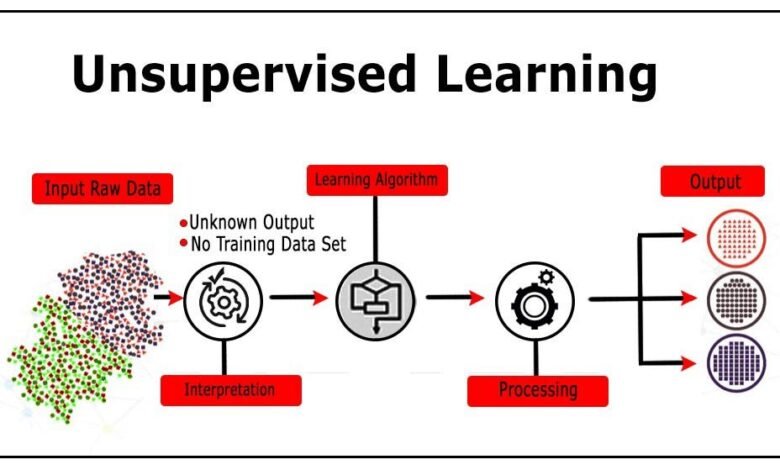
Unsupervised learning is a part of machine learning that trains on a dataset without labeled outputs. It finds hidden patterns or intrinsic structures within input data. It’s like a detective trying to solve a case using clues without a clear outcome.
The Core of UL: Key Concepts
To comprehend unsupervised learning, grasping the concept of features and clusters is crucial.
Features
Features are the measurable properties or characteristics of the phenomena being observed. They represent the dimensions or variables in the dataset.
Clusters
Clusters are groups of similar data points or features. Identifying these groups is known as clustering, an essential technique in ul.
Types of Unsupervised Learning
UL broadly falls under two categories: clustering and dimensionality reduction.
Clustering
Clustering entails grouping information factors in this manner so that information factors within the identical group (cluster) are more significant, much like every apart from the ones in different groups.
Dimensionality Reduction
Dimensionality discount reduces the variety of random variables below attention by acquiring a fixed primary variable. It is finished to simplify the dataset at the same time as preserving its crucial information.
It is done to simplify the dataset while retaining its essential information.
Unveiling UL: The Process
UL primarily involves four steps: data collection, data preparation, model training, and interpretation of results.
Data Collection
The initial step is gathering data relevant to the problem at hand.
Data Preparation
Data preparation involves cleaning and processing the data to make it suitable for analysis.
Model Training
During training, the algorithm identifies patterns and structures within the data.
Interpretation of Results
Interpreting results in unl can be challenging as there are no correct answers or labels. The validity of the results often relies on the interpretation of the domain experts.
Applications of UL
Unsupervised getting to know has a wide variety of applications: patron segmentation, anomaly detection, advice systems, and records visualization.
The Future of UL
Unsupervised mastering, even though challenging, has considerable potential. We can count on unsupervised mastering as computational abilities grow to play increasingly prominent positions in information exploration and understanding.
Conclusion
Unsupervised learning can uncover hidden patterns and systems in data, giving thrilling possibilities for advancing machine learning. As we preserve to refine those algorithms, the promise of actual knowledge of our complicated facts universe comes in the direction of reality.